*NEW* The Power Of Data Science And Predictive Analytics
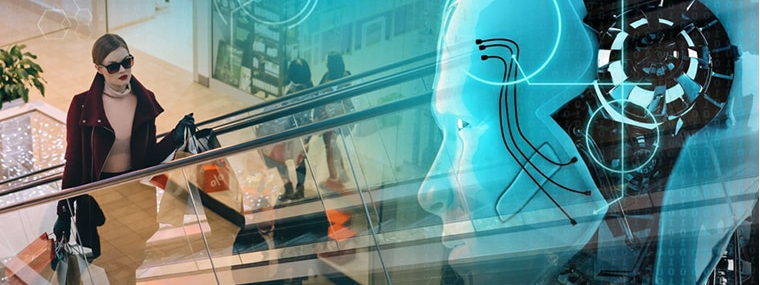
Data science and predictive analytics are becoming as essential a part of the retail arsenal as points of contact for consumers, and assistants on the ground.
As consumers become more technologically sophisticated, customer expectations grow ever higher. Today’s shoppers take as The New Normal the ability to research and compare goods or services online, contact their favourite brands from their mobile phones, use cutting edge technologies like augmented reality (AR) and virtual reality (VR) to refine their choices, make purchases online for delivery to their door, or order from home and pick up in store.
The back end operations that enable retailers to meet heightened customer demand require a data-enriched infrastructure, underpinning the entire business, sophisticated tools for analysing and extracting insights from this information, and a powerful and usually cloud-based system for delivering these insights throughout the organisation, to supply chain partners, and to other stakeholders.
All of these processes require an investment in digital technology - and many if not all of them become more powerful and efficient, through the use of data science and predictive analytics.
The Role Of Data Science And Predictive Analytics In Retail
Data science is a discipline one of whose main objectives is extracting meaningful insights from business processes and information. A variety of tools and analysis methods may be used, but the end result remains the same: finding trends and discovering ideas from data, and communicating those findings to people in the organisation who can then put them into action.
Especially when dealing with large volumes of information, such as the data sets routinely encountered by a retail organisation, text is often difficult for stakeholders to decipher. That’s why data science frequently employs visualisations such as graphs, pie charts, dashboards, and infographics to make its findings more accessible to readers from a non-technical background.
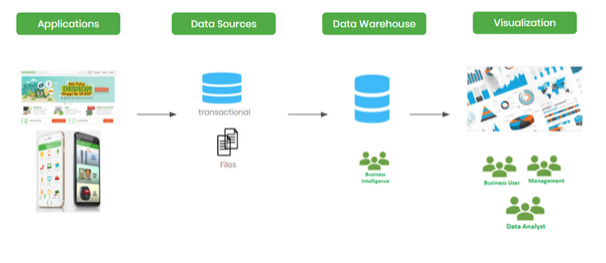
(Image source: Google)
As the name suggests, predictive analytics involves the study of information gathered historically or in real time, and the formulation of likely outcome scenarios, based on this analysis. These forecasts and any insights associated with them may be communicated to business stakeholders using the kinds of visualisation models and dashboards that data scientists routinely employ.
Like so many other industries, the retail sector is becoming increasingly data-dependent. Data science and predictive analytics offer retailers a number of options - both for streamlining and optimising their operations, and in forging improved and sustained relationships with their customers.
Data-Assisted Store Design
The actual physical location of a retail outlet is a major determinant of its level of success - both as a standalone entity, and as a contributor to the profitably of the organisation in general. To establish optimal siting, a simple data science algorithm enables analysts to explore customer information - with a specific eye on demographic factors. The algorithm can make logical connections between customer data, ZIP or postal codes, historical demand, and the retailer’s existing store network, to determine the best place to locate new stores.
Once construction is complete, information generated by in-store cameras and sensors can add to existing data sources such as transaction histories and customer surveys, to yield insights that enable retailers to discover new buying patterns and customer behaviours - which can enable them in turn to adjust interior design and displays, to capitalise on selling opportunities.
Enabling Real-Time Response And Sustained Customer Relationships
With so much of retail now occurring in an omni-channel environment, organisations must keep track of what’s going on in their network at numerous levels and touch points. For this reason, edge computing technologies are gaining ground, with their ability to put data gathering and analysis powers close to where interactions and transactions are actually occurring.
Gartner, Inc., predicts that by 2022, 75 percent of the information generated by enterprises will be created and processed outside of traditional data centres, or the cloud. The Internet of Things or IoT is expected to have a big role in this growth, with retailers routinely deploying embedded sensors in shop floors, on shopping carts, shelves, and as wearable tags for employees. Global Market Insights expects the retail IoT market to reach over $30 billion by 2024.
These technologies can also make it possible for retailers to respond to the results of data analysis in place, and in real time, without network latency or bandwidth issues - a real plus for enabling rapid response to customer demands. Personalised experiences on digital displays, real-time inventory management for click-and-collect, in-store navigation, and tailored promotions may also be facilitated.
Detecting Fraud And Analysing Warranty Claims
Protecting the organisation and its customers from reputational damage and financial losses due to fraud remains a top priority. Fraud detection algorithms may employ deep neural networks to provide continuous monitoring, and predictive analytics to forecast future activities based on existing patterns of fraudulent behaviour on the part of perpetrators.
Warranty analysis is an important aspect of fraud monitoring, and involves data and text mining to yield deeper insights into claims patterns and problem areas. This complex methodology concentrates on detecting anomalies in warranty claims.
Setting Prices And Making Market Predictions
Price optimisation routines take into consideration the costs to produce an item, the spending power of a typical consumer, and the price offered by competitors in the same retail space. Data is typically gathered from multiple channels to assess the overall situation regarding price flexibility, with factors such as location, customer buying habits, and seasonal price fluctuations also taken into account.
At a larger scale, market basket analysis uses the existing pool of customer transactions to generate predictions about the future state of the market. Such predictive analytics models usually run through a rule mining algorithm, and enable retailers to make future decisions concerning the business, at scale.
Inventory Management And Supply Chain Optimisation
For inventory management, powerful machine learning algorithms and data analytics platforms can detect patterns and correlations between the various elements in stock, and their relevant supply chains. Algorithms are typically constructed with a flexibility that allows them to identify patterns of high or low demand, and develop optimised strategies for emerging sales trends, delivery, and stock management.
Data science and data engineering platforms can also unlock the insights buried in log, sensor, and machine data. File servers, plant machinery, customer-owned appliances, cell towers, energy grid infrastructure, and product logs may also yield valuable information.
With data engineering tools, structured data such as geo-location, public records, Customer Relationship Management (CRM), Enterprise Resource Management (ERP), and mainframe, may combine with unstructured data (such as information from sensors and logs) to provide insights about trends, patterns, and anomalies that can improve decisions, drive better operations and supply chain performance, and save revenue.